Part II of the series: Music meets high-tech – How Georgian folk music advances complex algorithmics
Without technological foundations, the boldest and most ingenious ideas remain castles in the air. In this instalment of our tour through the AudioLabs, we meet the master builders of Music Signal Analysis: Meinard Müller’s team at the AudioLabs researches the foundations of audio signal processing. They test modern technologies of signal analysis and pattern recognition and advance them further by applying them to challenging music scenarios.
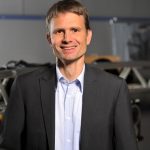
The professor and chair of Semantic Audio Signal Processing has been part of the AudioLabs team since September 2012. His main research focus is the development of methods for analyzing acoustic signals and computer-based music processing (Music Information Retrieval – MIR), that is, the analysis of audio signals in music. Such signals have specific characteristics, including melody, timbre, and rhythm. They are already used widely today, to sort digital music libraries by genre or to find similar-sounding songs, for instance.
Müller is a mathematician and computer scientist and is particularly interested in interdisciplinary research: Music may be the motivation and context for his work, “but the focus is on signal processing, pattern recognition, and algorithms,” he says. Time and time again, he discovers cross-disciplinary scenarios, such as the computer-based analysis of famous operas or Georgian folk songs, which are part of the UNESCO World Cultural Heritage. In the latter case, Müller joined forces with Frank Scherbaum and Georgian ethnomusicologists to research tonal aspects of these polyphonic songs. “Of course, this includes many projects that are surprising at first glance. But these scenarios are far removed from the standard and therefore particularly interesting,” Müller explains. Such unconventional cases are used to test how theoretical considerations can be translated into practice and to test the algorithms’ boundaries. “Nevertheless, this is complex algorithmics we are doing here,” the researcher, whose findings are used to consider problems from multiple angles, emphasizes.
His penchant for music is just as crucial to his work as his great thirst for knowledge. Even before graduating school, he acquired some basic knowledge of programming and read computer science in addition to mathematics in Bonn. Although his focus had already shifted to computer science during his doctorate, his work benefits from the math knowledge he acquired. “For my current work, these basics are essential, so these studies were the perfect training,” he explains. After a stint as postdoctoral researcher at Keio University in Japan, his former doctoral supervisor inspired him to look into signal and music processing – and in particular at digital music libraries. After his postdoc in Bonn and a research position at the Max Planck Institute for Computer Science in Saarbrücken, his path finally led him to Erlangen and the AudioLabs, where he and his team are currently working on Deep Learning methods. For them, it is particularly important to identify the causes of problems. Deep Learning often delivers good results but requires a large amount of well-annotated training data. However, these are often not available for special scenarios and applications, such as the analysis of Georgian chants. Nevertheless, theses are researched using classical methods and Deep Learning, to develop reliable analysis methods. After this, the results of the working methods are compared, differences are analyzed, and advantages as well as disadvantages are worked out for each case. “We also want to find out how different processes can benefit from each other. For example, the flexibility of data-driven methods can often be incorporated quite well into model-based processes, while the conventional methods provide the required knowledge,” Müller says.
His enthusiasm for training young scientists also helps in the continuous development of his research field. “I want people to learn the basic techniques from me and understand how methods can be transferred or why some algorithms just don’t work.” In return, he constantly receives new impulses from PhD students: “The tools are always changing. Nowadays, young people primarily work with the Python programming language, which is something I had to learn myself, just like Deep Learning itself,” he emphasizes. This is also one of the big advantages Müller sees in the interaction between the AudioLabs and the Fraunhofer IIS: He has first-hand insights into which methods are relevant for the industry and can teach students and doctoral candidates the mathematical and technnological basics while advancing them together. This also benefits the IIS, because it gives employees additional opportunities to expand their skills, complete doctorates, and try out new methods. In turn, the fundamentals and different perspectives they learn at the AudioLabs help them approach IIS-projects in a flexible way. Overall, the researcher sees the AudioLabs as a “kind of bracket that connects industry and research.” He is enthusiastic about the continuous exchange with his colleagues, with whom he shares the fascination with audio and signal processing topics.
Even outside the walls of the AudioLabs and the Fraunhofer IIS, Müller is committed to the exchange of knowledge. In 2020/21, he was the President of the International Society of Music Information Retrieval (ISMIR), and in 2020, the Institute of Electrical and Electronics Engineers (IEEE) named him an IEEE Fellow for his work in the field of music signal processing – an honor awarded to only a few members. The exchange with the professional world is high on the priority list, because for him, there is always something new to discover.
Have you already seen part I of our tour?
Header image: © AudioLabs; Meinard Müller, Christian Dittmar